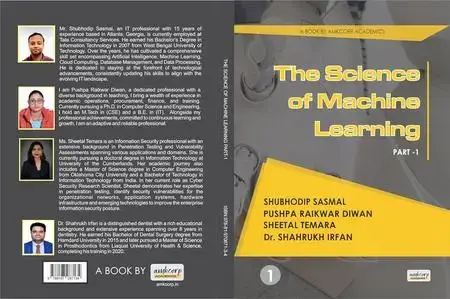
Free Download The Science of Machine Learning by SHUBHODIP SASMAL, PUSHPA RAIKWAR DIWAN, SHEETAL TEMARA
English | March 17, 2024 | ISBN: N/A | ASIN: B0CW17VCLS | 212 pages | PDF | 30 Mb
The book titled "The Science of Machine Learning Part 1" serves as a comprehensive guide for both beginners and experienced practitioners in the field of machine learning. Covering a wide range of topics, the book provides a thorough introduction to the fundamentals of machine learning, as well as advanced techniques and emerging trends.
In the first chapter, readers are introduced to the concept of machine learning, its historical context, and its importance and applications in various domains. The chapter also explores different types of machine learning and addresses key terminologies, challenges, and limitations associated with the field.
The second chapter delves into the mathematical foundations essential for understanding machine learning algorithms. Topics covered include linear algebra, probability and statistics, calculus, optimization techniques, information theory basics, and numerical methods and algorithms.
Moving forward, the book explores data preprocessing and exploration techniques in Chapter 3. Readers learn about data cleaning, handling missing values, feature scaling, normalization, feature engineering, exploratory data analysis (EDA), data visualization, and dimensionality reduction methods.
Chapters 4 and 5 focus on supervised and unsupervised learning algorithms, respectively. Readers are introduced to popular algorithms such as linear regression, logistic regression, decision trees, random forests, support vector machines (SVM), k-nearest neighbors (k-NN), and ensemble methods for supervised learning, as well as k-means clustering, hierarchical clustering, Gaussian mixture models (GMM), principal component analysis (PCA), t-distributed stochastic neighbor embedding (t-SNE), and association rule learning for unsupervised learning.
Chapter 6 explores neural networks and deep learning, covering topics such as artificial neural networks (ANN), convolutional neural networks (CNN), recurrent neural networks (RNN), long short-term memory (LSTM), and generative adversarial networks (GAN).
In Chapter 7, readers learn about model evaluation and validation techniques, including cross-validation, performance metrics, bias-variance tradeoff, hyperparameter tuning, model interpretability, and explainability.
Leave a Reply
You must be logged in to post a comment.