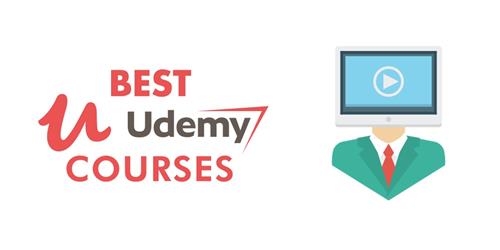
Free Download Data Science – Supervised Machine Learning in Python
Last updated 11/2024
MP4 | Video: h264, 1920×1080 | Audio: AAC, 44.1 KHz
Language: English | Size: 2.05 GB | Duration: 6h 24m
Full Guide to Implementing Classic Machine Learning Algorithms in Python and with Scikit-Learn
What you’ll learn
Understand and implement K-Nearest Neighbors in Python
Understand the limitations of KNN
User KNN to solve several binary and multiclass classification problems
Understand and implement Naive Bayes and General Bayes Classifiers in Python
Understand the limitations of Bayes Classifiers
Understand and implement a Decision Tree in Python
Understand and implement the Perceptron in Python
Understand the limitations of the Perceptron
Understand hyperparameters and how to apply cross-validation
Understand the concepts of feature extraction and feature selection
Understand the pros and cons between classic machine learning methods and deep learning
Use Sci-Kit Learn
Implement a machine learning web service
Requirements
Python, Numpy, and Pandas experience
Probability and statistics (Gaussian distribution)
Strong ability to write algorithms
Description
In recent years, we’ve seen a resurgence in AI, or artificial intelligence, and machine learning.Machine learning has led to some amazing results, like being able to analyze medical images and predict diseases on-par with human experts.Google’s AlphaGo program was able to beat a world champion in the strategy game go using deep reinforcement learning.Machine learning is even being used to program self driving cars, which is going to change the automotive industry forever. Imagine a world with drastically reduced car accidents, simply by removing the element of human error.Google famously announced that they are now "machine learning first", meaning that machine learning is going to get a lot more attention now, and this is what’s going to drive innovation in the coming years. It’s embedded into all sorts of different products.Machine learning is used in many industries, like finance, online advertising, medicine, and robotics.It is a widely applicable tool that will benefit you no matter what industry you’re in, and it will also open up a ton of career opportunities once you get good.Machine learning also raises some philosophical questions. Are we building a machine that can think? What does it mean to be conscious? Will computers one day take over the world?In this course, we are first going to discuss the K-Nearest Neighbor algorithm. It’s extremely simple and intuitive, and it’s a great first classification algorithm to learn. After we discuss the concepts and implement it in code, we’ll look at some ways in which KNN can fail.It’s important to know both the advantages and disadvantages of each algorithm we look at.Next we’ll look at the Naive Bayes Classifier and the General Bayes Classifier. This is a very interesting algorithm to look at because it is grounded in probability.We’ll see how we can transform the Bayes Classifier into a linear and quadratic classifier to speed up our calculations.Next we’ll look at the famous Decision Tree algorithm. This is the most complex of the algorithms we’ll study, and most courses you’ll look at won’t implement them. We will, since I believe implementation is good practice.The last algorithm we’ll look at is the Perceptron algorithm. Perceptrons are the ancestor of neural networks and deep learning, so they are important to study in the context of machine learning.One we’ve studied these algorithms, we’ll move to more practical machine learning topics. Hyperparameters, cross-validation, feature extraction, feature selection, and multiclass classification.We’ll do a comparison with deep learning so you understand the pros and cons of each approach.We’ll discuss the Sci-Kit Learn library, because even though implementing your own algorithms is fun and educational, you should use optimized and well-tested code in your actual work.We’ll cap things off with a very practical, real-world example by writing a web service that runs a machine learning model and makes predictions. This is something that real companies do and make money from.All the materials for this course are FREE. You can download and install Python, Numpy, and Scipy with simple commands on Windows, Linux, or Mac.This course focuses on "how to build and understand", not just "how to use". Anyone can learn to use an API in 15 minutes after reading some documentation. It’s not about "remembering facts", it’s about "seeing for yourself" via experimentation. It will teach you how to visualize what’s happening in the model internally. If you want more than just a superficial look at machine learning models, this course is for you."If you can’t implement it, you don’t understand it"Or as the great physicist Richard Feynman said: "What I cannot create, I do not understand".My courses are the ONLY courses where you will learn how to implement machine learning algorithms from scratchOther courses will teach you how to plug in your data into a library, but do you really need help with 3 lines of code?After doing the same thing with 10 datasets, you realize you didn’t learn 10 things. You learned 1 thing, and just repeated the same 3 lines of code 10 times…Suggested Prerequisites:calculus (for some parts)probability (continuous and discrete distributions, joint, marginal, conditional, PDF, PMF, CDF, Bayes rule)Python coding: if/else, loops, lists, dicts, setsNumpy, Scipy, MatplotlibWHAT ORDER SHOULD I TAKE YOUR COURSES IN?:Check out the lecture "Machine Learning and AI Prerequisite Roadmap" (available in the FAQ of any of my courses, including the free Numpy course)UNIQUE FEATURESEvery line of code explained in detail – email me any time if you disagreeNo wasted time "typing" on the keyboard like other courses – let’s be honest, nobody can really write code worth learning about in just 20 minutes from scratchNot afraid of university-level math – get important details about algorithms that other courses leave out
Overview
Section 1: Introduction and Review
Lecture 1 Introduction and Outline
Lecture 2 How to Succeed in this Course
Lecture 3 Where to get the Code and Data
Lecture 4 Review of Important Concepts
Section 2: K-Nearest Neighbor
Lecture 5 K-Nearest Neighbor Intuition
Lecture 6 K-Nearest Neighbor Concepts
Lecture 7 KNN in Code with MNIST
Lecture 8 When KNN Can Fail
Lecture 9 KNN for the XOR Problem
Lecture 10 KNN for the Donut Problem
Lecture 11 Effect of K
Lecture 12 KNN Exercise
Lecture 13 Suggestion Box
Section 3: Naive Bayes and Bayes Classifiers
Lecture 14 Bayes Classifier Intuition (Continuous)
Lecture 15 Bayes Classifier Intuition (Discrete)
Lecture 16 Naive Bayes
Lecture 17 Naive Bayes Handwritten Example
Lecture 18 Naive Bayes in Code with MNIST
Lecture 19 Non-Naive Bayes
Lecture 20 Bayes Classifier in Code with MNIST
Lecture 21 Linear Discriminant Analysis (LDA) and Quadratic Discriminant Analysis (QDA)
Lecture 22 Generative vs Discriminative Models
Section 4: Decision Trees
Lecture 23 Decision Tree Intuition
Lecture 24 Decision Tree Basics
Lecture 25 Information Entropy
Lecture 26 Maximizing Information Gain
Lecture 27 Choosing the Best Split
Lecture 28 Decision Tree in Code
Section 5: Perceptrons
Lecture 29 Perceptron Concepts
Lecture 30 Perceptron in Code
Lecture 31 Perceptron for MNIST and XOR
Lecture 32 Perceptron Loss Function
Section 6: Practical Machine Learning
Lecture 33 Hyperparameters and Cross-Validation
Lecture 34 Feature Extraction and Feature Selection
Lecture 35 Comparison to Deep Learning
Lecture 36 Multiclass Classification
Lecture 37 Sci-Kit Learn
Lecture 38 Regression with Sci-Kit Learn is Easy
Section 7: Building a Machine Learning Web Service
Lecture 39 Building a Machine Learning Web Service Concepts
Lecture 40 Building a Machine Learning Web Service Code
Section 8: Conclusion
Lecture 41 Whatâs Next? Support Vector Machines and Ensemble Methods (e.g. Random Forest)
Section 9: Setting Up Your Environment (FAQ by Student Request)
Lecture 42 Pre-Installation Check
Lecture 43 Anaconda Environment Setup
Lecture 44 How to install Numpy, Scipy, Matplotlib, and Sci-Kit Learn
Section 10: Extra Help With Python Coding for Beginners (FAQ by Student Request)
Lecture 45 How to Code by Yourself (part 1)
Lecture 46 How to Code by Yourself (part 2)
Lecture 47 Proof that using Jupyter Notebook is the same as not using it
Lecture 48 Python 2 vs Python 3
Section 11: Effective Learning Strategies for Machine Learning (FAQ by Student Request)
Lecture 49 How to Succeed in this Course (Long Version)
Lecture 50 Is this for Beginners or Experts? Academic or Practical? Fast or slow-paced?
Lecture 51 Machine Learning and AI Prerequisite Roadmap (pt 1)
Lecture 52 Machine Learning and AI Prerequisite Roadmap (pt 2)
Section 12: Appendix / FAQ Finale
Lecture 53 What is the Appendix?
Lecture 54 BONUS
Students and professionals who want to apply machine learning techniques to their datasets,Students and professionals who want to apply machine learning techniques to real world problems,Anyone who wants to learn classic data science and machine learning algorithms,Anyone looking for an introduction to artificial intelligence (AI)
Homepage
www.udemy.com/course/data-science-supervised-machine-learning-in-python/
Buy Premium From My Links To Get Resumable Support,Max Speed & Support Me
Rapidgator
fefrh.Data.Science.Supervised.Machine.Learning.in.Python.part2.rar.html
fefrh.Data.Science.Supervised.Machine.Learning.in.Python.part3.rar.html
fefrh.Data.Science.Supervised.Machine.Learning.in.Python.part1.rar.html
Fikper
fefrh.Data.Science.Supervised.Machine.Learning.in.Python.part1.rar.html
fefrh.Data.Science.Supervised.Machine.Learning.in.Python.part2.rar.html
fefrh.Data.Science.Supervised.Machine.Learning.in.Python.part3.rar.html
Data Science – Supervised Machine Learning in Python Torrent Download , Data Science – Supervised Machine Learning in Python Watch Free Online , Data Science – Supervised Machine Learning in Python Download Online